Bayesian-based flood damage assessment
According to an analysis by the International Flood Initiative, the number of water-related disasters has increased from more than 50 to more than 150 events per year since 1992. Each year, approximately 25,000 people lose their lives, more than 500 million people lose their livelihoods and property, and the global economy loses more than $60 billion. This does not include the loss of cultural heritage and natural resources.
According to historical data, the number of natural disasters increased from an average of 4.1 per year in the 30-year period from 1958 to 1987 to 7.8 per year in the 30-year period from 1988 to 2017. In terms of disaster types, typhoons accounted for 66%, floods for 22%, earthquakes for 8%, and other types of disasters for 4%, indicating that the main types of disasters in Taiwan are hydrometeorological disasters (typhoons, floods), in line with the global trend.

In this study, Bayesian theory is used as a framework to develop an economic loss model for rapid flooding. As shown in the figure above, the relationship between the influence factors such as rainfall, inundation depth, and surface slope and the loss (i.e., rloss) is established, and then a rapid assessment of the potential economic loss is made based on the forecast data of different events, which is helpful for the reference of the disaster response strategy. Special thanks to the Helmholtz Centre Potsdam GFZ German Research Centre for Geosciences for providing the HOWAS21 flood damage database. Using the loss data from this database, this study can investigate the effects of the influencing factors on the loss using three methods, including (1) the depth-damage curve, (2) the Bayesian regression method, and (3) the Bayesian network. This study focuses on the application of these models for flood damage assessment in industrial areas.
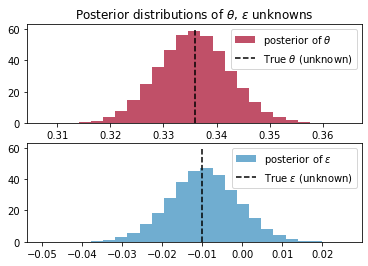
The figure above is a preliminary result of the first method, the depth-damage method, which assumes that the damage is only related to the depth of inundation, and then uses the preset parameters along with the depth-damage equation to generate artificial data. These data are then used to find the corresponding two parameters andby Bayesian inference. From the results, it can be seen that the highest probability density overlaps with the preset parameter values, which also demonstrates the ability of Bayesian to infer the parameters in the depth-damage equation.